Generative AI vs. Predictive AI: What’s the Difference?
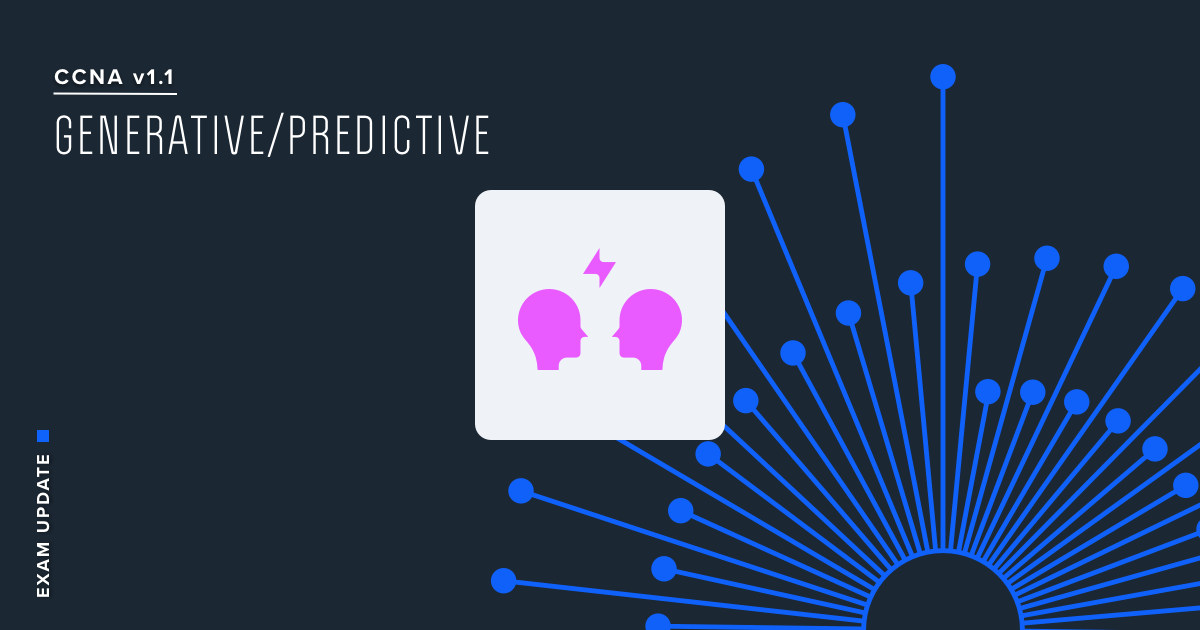
Quick Definition: Generative AI creates new and unique content by learning from data patterns, while predictive AI analyzes data to predict what will happen next.
AI is changing the game in just about every field—health, finance, entertainment, you name it. As AI keeps advancing, it is important to classify it into various forms to understand each type's strengths and limitations. Two key types are generative AI and predictive AI. If you're gearing up for the updated Cisco CCNA v1.1 exam, you'll need to understand both generative and predictive AI and their roles in network operations. Don’t worry—we’ve got your back. In this article, we’ll quickly dive into what generative and predictive AI are, how they’re different, where they’re used, and some of the challenges they bring.
What is Generative AI?
Generative AI is a type of artificial intelligence that creates new content, including text, images, and music, by learning from existing data patterns. While ‘regular’ types of AI could work on data or make forecasts, new generative AI can create text, visuals, music, and even a new virtual reality. Consider it as the artist of the AI family, as it never stops experimenting and finding new patterns.
One key feature of generative AI is its ability to create entirely new data sets, such as realistic images of nonexistent people or music that sounds human-made. This makes it valuable in areas like art, entertainment, and marketing, where it generates content for users.
Uses of Generative AI
Here are some common ways generative AI is being used:
Art Generation: Generative AI can create original artwork by learning from existing art styles and techniques, producing new pieces in various formats, from paintings to digital designs.
Synthetic Data: Generative AI generates artificial data that mimics real-world data, which is useful for training machine learning models, testing systems, and maintaining privacy by using non-sensitive data.
Virtual Reality and Gaming: Generative AI helps design immersive environments, characters, and scenarios in virtual reality and gaming.
Product Design and Prototyping: Generative AI can generate multiple design variations, optimize product features, and reduce the time and cost associated with traditional design methods.
Personalized Content: Generative AI can tailor content, such as personalized ads, music playlists, or articles, based on what it learns about individual user preferences and behavior.
Network Operations: Generative AI can create realistic data for training, design network architectures, and set up experiments to fine-tune network performance and reliability.
What is Predictive AI?
Predictive AI is like a real-world 'crystal ball.' Instead of creating new content, it analyzes data to forecast future trends and outcomes. This kind of AI is common in industries such as finance to predict market trends or in the healthcare industry to predict patient outcomes.
The real power of predictive AI lies in how quickly and accurately it can crunch massive amounts of data. Predictive AI uses more complex algorithms, such as machine learning, and can be highly precise.
This capability helps businesses make better decisions, streamline business procedures, and provide a competitive advantage. Predictive AI is widely used in a wide range of applications, from predicting customers’ behavior to forecasting sales and managing risk.
Uses of Predictive AI
Here are some common use cases of predictive AI:
Forecasting: Predictive AI analyzes past data to forecast future events, like sales trends, weather patterns, or stock market movements.
Risk Assessment: Predictive AI evaluates potential risks and predicts possible outcomes, which is crucial for industries like finance and insurance to assess credit risk, detect fraud, and ensure investment safety.
Recommendation Systems: By analyzing user data, predictive AI powers recommendation engines that suggest products, services, or content tailored to individual preferences.
Healthcare Predictions: In healthcare, predictive AI can help forecast patient outcomes, such as disease progression, treatment responses, and hospital readmission rates, allowing healthcare providers to offer more personalized care.
Customer Behavior Analysis: Predictive AI can predict customer churn, retention rates, and purchasing behavior, helping businesses enhance customer segmentation and targeting strategies.
Operational Efficiency: Predictive AI can improve operations by forecasting demand, managing inventory, and improving resource allocation.
Network Operations: In network management, predictive AI is key for traffic modeling, spotting potential bottlenecks, anticipating equipment failures, and enhancing network control and protection.
What are the Key Differences Between Generative AI and Predictive AI?
The biggest difference between generative AI and predictive AI lies in what they produce. Generative AI is all about creating something new, like a writer crafting a story or a composer writing music. Predictive AI, on the other hand, is like a fortune teller, using existing data to predict future events, whether it’s stock market trends or customer behavior.
Differences in Methodologies and Algorithms Used
Generative AI creates new data sets using techniques like Generative Adversarial Networks (GANs) and Variational Autoencoders (VAEs). Predictive AI makes forecasts using statistical methods like regression, decision trees, and neural networks.
In short, generative AI is focused on generating new data, while predictive AI is focused on forecasting based on existing data.
Variations in Data Requirements and Processing Techniques
The second major difference is a bit subtle and relates to data requirements and methods applied to the data. Generative AI usually requires large datasets for training and uses intensive processes to define patterns and styles for creating realistic content.
Predictive AI, on the other hand, can work with smaller data sets and is designed to prioritize accuracy in forecasts over content generation. This difference in data handling shapes the type of data they produce and how they’re used.
Industry-Specific Applications of Generative AI
Ever wondered how video games provide such an interesting environment or how applications come up with content suggestions? That is what can be referred to as the generative AI at work!
In entertainment, generative AI is being used to create realistic characters and immersive environments that draw audiences in. Marketing teams are leveraging its ability to craft catchy phrases, taglines, and personalized promotional messages, even automating email newsletters to reach clients more effectively.
In the realm of network operations, generative AI is a powerful tool for designing and testing new network configurations, as well as modeling and predicting network scenarios to enhance reliability and performance.
Industry-Specific Applications of Predictive AI
Perhaps you want to know more about how enterprises make forecasts or allocate risks. Enter Predictive AI! In finance, it can be used to predict stock prices and assess credit risk or credit score. It can also be used in operations to forecast customer demand and in supply chain product management. Additionally, predictive AI is valuable in healthcare, where it can forecast the impact of a specific treatment or evaluate the usage of medical facilities.
Other uses of predictive AI include sports, where it can provide insights into the performance of players. In network operations, predictive AI can help forecast network traffic, recognize risks in network security, and direct the distribution of resources in an attempt to avoid network load and failure. It's also worth noting the syllabus of the CCNA exam now includes understanding predictive AI.
Challenges for Predictive and Generative AI
Like with any new technology, AI has its drawbacks. Generative AI, for example, requires vast data sets and significant computational resources to create high-quality content. Ethically, there’s a risk of misuse, like creating deep fakes or spreading fake news, so it’s crucial to manage its use responsibly. Despite these issues, the potential benefits make addressing them worthwhile.
Predictive AI also has its challenges, such as needing clean and accurate data for reliable predictions, which can be hard to obtain. There’s also the risk of biased predictions if the training data is unbalanced, leading to unfair outcomes. Balancing accuracy with fairness is a key issue, but overcoming these challenges is essential because predictive AI provides valuable insights and forecasting capabilities.
Considerations for Choosing Between Generative and Predictive AI
Deciding between generative and predictive AI ultimately comes down to the specific needs of your project. If your focus is on creating new content or products—whether it’s text, images, or experiences—generative AI is your best bet.
On the other hand, if your project involves analyzing trends or making critical business decisions, predictive AI is better suited for the task. It’s designed to sift through data, identify patterns, and forecast outcomes. Your choice will depend on your project and the type of data you have or aim to work with. By doing so, you can harness the full potential of AI.
Want to get started with CBT Nuggets CCNA Course? Get a free 7-day trial.
delivered to your inbox.
By submitting this form you agree to receive marketing emails from CBT Nuggets and that you have read, understood and are able to consent to our privacy policy.